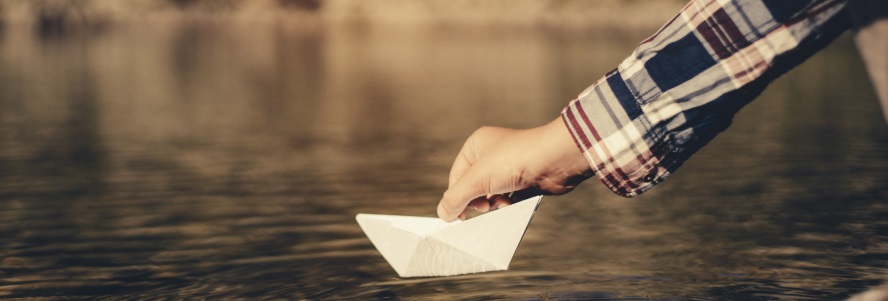
Big data, smart data, data lakes, data warehouses, data repositories! It seems like nowadays, the word “data” makes an appearance at every sales conference and in every article. Though this article’s no exception, it’s a little different: we’re here to help you understand these oft-confusing terms and find your way to the right solution.
Most organizations know what they want out of their sales operations, but they often struggle to transform that vision into a reality.
That’s especially tricky to achieve when you need to process large volumes of data from multiple sources. Cleaning, centralizing, validating, and analyzing data are all highly technical and intensive tasks, but the value they can bring to your company is immense.
Data storage: the first stop, but not the destination
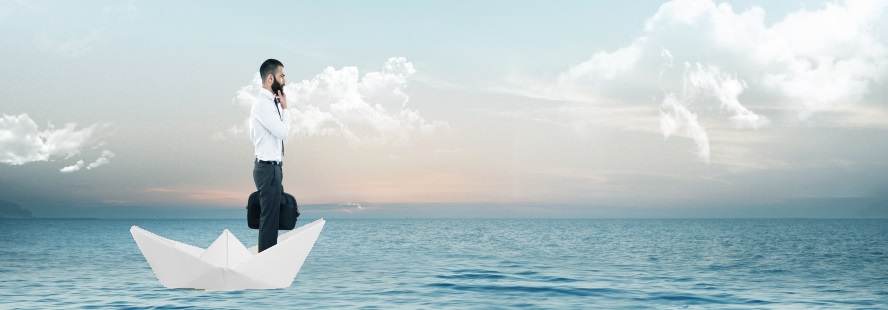
A data lake is, in effect, a repository that allows you to store both structured and unstructured data at any scale. The main advantage of this architecture is that the data can be used in its “natural format” – i.e., without having to be structured first – for the purposes of processing, analytics, and visualizations.
Data swamps, meanwhile, are damaged data lakes that either are inaccessible to their potential audience or cannot provide any valuable information. In other words, a data swamp is a data lake “gone bad.” The line between data lakes and data swamps can be a thin one, especially since there’s a relatively low number of users who can realize the full benefits of data lakes.
While undeniably popular, neither of these concepts is particularly new or revolutionary. To perform proper data management, you’ll have to concentrate on both structure and format, which brings us to another highly used term in the data world.
One of the most common ways of storing large volumes of data, data warehouses are essentially massive repositories of integrated data drawn from one or multiple sources. Warehouses can store both current and historical data; they’re used to create reports for both sales reps and management, but also for analytics and similar operations.
In contrast to data lakes, information isn’t “thrown” into a warehouse; rather, it’s transformed, structured, and assigned a specific purpose (say, a particular business area).
Where lakes typically need an expert hand to be useful, warehouses are typically either semi- or fully automated – offering easier access for the common user as well as for company leaders who want to analyze sales figures and related information.
Your North Star: data processing
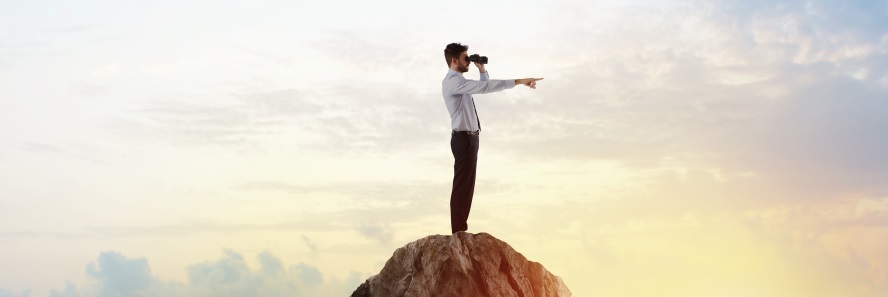
The debate over the merits of data warehouses vs. data lakes is difficult to settle. In our experience, though, the most important detail is not your storage methodology, but the way you process your data.
For example, one of our largest clients is a national telecommunications company, and they came to us with an enormous amount of data to process. What architecture recommendation did we make for them? None at all. Although we did ultimately utilize a flexible storage solution, that wasn’t one of our prime considerations.
Our client’s business units required the permanent processing of many terabytes of data that had been drawn from multiple sources in multiple formats. The large volume of kickouts and the consequently low quantity of valid data made both storage and usage into major issues.
The first step was establishing best practices for processing the data. It was no problem to replace our client’s legacy systems and combine their formerly disparate data sources into a single repository. However, cleanliness, not storage, was our main concern – so once we got the data in one place, we evaluated it for purity and prioritized it accordingly with logical algorithms.
With the data centralized, the next step was to make it widely accessible through our easy-to-use, code-free data management.
Suddenly, our client’s salespeople had instant access to information about customers and prospects, while management gained the power to execute accurate sales planning. This repository ultimately came to be recognized as a single source of truth for the company’s multiple business units – enabling the creation of better metrics along the way.
The point is, despite storage type and general architecture being common points of contention, they were in fact the least of our client’s problems – and they should be the least of yours, too. No matter how you’re keeping your data, if your reps and managers don’t have easy, real-time access to digestible information, all your investments in storage will be moot!
Data transformation: the wind in your sails
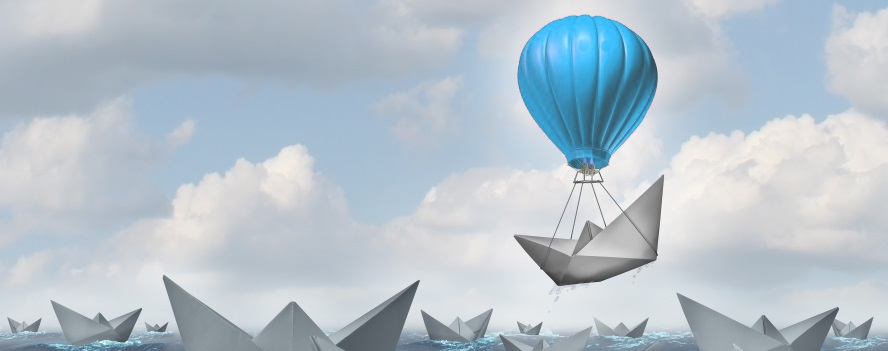
The concept of a single source of truth for corporate data is gaining wide appeal. However, just as a lake isn’t much good if the water is contaminated, a data repository can’t help you much if the data hasn’t been transformed into a usable format.
As many businesses have discovered, the data ocean’s perils don’t stop at processing. Even if you do have a flexible storage solution and know precisely what data is going through your system, it will eventually become obsolete. No matter which route you’re taking, new layers of information will be added constantly, making your data run deeper and deeper.
So, how can you stay afloat?
Well, instead of pushing against the current, use it to your advantage. Consolidate disparate information into a single platform so that you can analyze your data and use it as a catalyst for sales enablement. In other words, strategically transform your data into the wind that fills your sails (or sales!).
It’s great to see companies starting to discover the potential of a single source of truth – after all, we’ve been talking about it and doing it for years. However, making the best of your data requires turning it into actionable insights that can not only improve your reps’ performance, but also give you a whole new perspective on the sales organization.
Those deep and meaningful analytics are the lighthouse that helps you make port in a storm of ever-shifting data.
With our no-code Data Repository and ETL capabilities, Optymyze can handle even the most complex data management as well as extraction, transformation, and load (ETL) requirements – managing and processing thousands of data tables with hundreds of millions of records that comprise multiple terabytes of data.